Publication reviewed:
Community water fluoridation predicts increase in age-adjusted and prevalence of diabetes in 22 states from 2005 and 2010
Fluegge K. – Journal of Water and Health. 2016;14(5):864-77
A Muddle of Models
OVERVIEW
The paper about fluoride and diabetes by Fluegge (J Water and Health 2016) examined county level estimates of diabetes prevalence and incidence and their association with community water fluoridation status at the county level in the United States in 2005 and 2010. Several statistical models were presented and the author concluded that the type of chemical used in the fluoridation process had an important role in predicting the incidence and prevalence of diabetes in a county. This is an awkward conclusion because the chemical formulation used to fluoridate a water system to an optimal level does not impact on the bioavailability of fluoride in consumer tap water.1-5 A further look into the methodology that comprised the statistical modeling reveals several key problems.
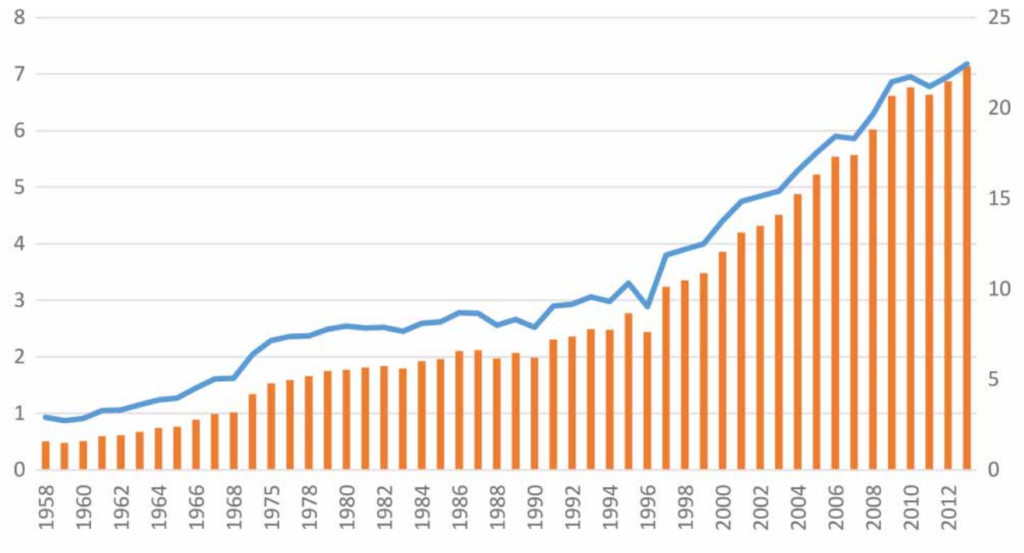
BASIC STATISTICAL MODELING ARCHITECTURE EMPLOYED
The basic framework of the analysis makes use of generalized estimating equations (GEE). This approach has been devised to address a key assumption in the world of generalized linear modeling: the need for independence of observations. GEE allows an investigator to examine multiple observations that may be correlated and thereby impair the assumption of statistical independence. So in longitudinal studies, GEE allows an investigator to make full use of data that is collected over time for the same study subject, by modeling the correlation within a subject and making an adjustment to the parameter estimate to account for the lack of independence. GEE allows the investigator to use one model to estimate the effect of the independent variables on multiple outcomes. In this case, Fluegge used the GEE approach to model county-level estimates of both incidence and prevalence of diabetes.
COUNTY-LEVEL ESTIMATION OF DIABETES
The incidence and prevalence estimates for diabetes were developed by the US Centers for Disease Control and Prevention (CDC) based on self-reported information collected in a telephone survey, the Behavioral Risk Factor Surveillance System (BRFSS).6,7 To get county-level estimates of diabetes incidence and prevalence of diabetes for every county in the US, CDC does something known as Small Area Estimation. Because of sampling limitations and costs, not all counties have robust data to make a sound estimate from the BRFSS. Using socio-demographic county data, CDC estimates what incidence and prevalence would be given the socio-demographic profile of counties where robust data exist. This allows data from the BRFSS to give an estimate to each county based on data from the US Census Bureau on age, sex, race, and Hispanic origin.
Here is how the CDC describes the method: “The county-level estimates for the over 3,200 counties or county equivalents (e.g., parish, borough, municipality) in the 50 US states, Puerto Rico, and the District of Columbia (DC) were based on indirect model-dependent estimates using Bayesian multilevel modeling techniques.8 This model-dependent approach employs a statistical model that “borrows strength” in making an estimate for one county from BRFSS data collected in other counties. Multilevel Poisson regression models with random effects of demographic variables (age 20–44, 45–64, ≥65; race; sex) at the county-level were developed. State was included as a county-level covariate.”8
The accuracy of self-reported diabetes and the ability to estimate incidence using the BRFSS also has limitations. See additional note section below.
ESTIMATING FLUORIDE EXPOSURE
Fluegge used several variables to create measures of fluoride exposure. The intent seems to be to create a county-level profile of fluoride exposure for the public water systems in a county, However, the use of multiple variables for fluoride exposure in the statistical model make the regression coefficients difficult to interpret since a given county will be characterized by several variables that are linked together. This is known as effect modification. In the Fluegge model, there are variables for “amount of added fluoride”, “fluoridation chemical” and “years of fluoridation”. To interpret the statistical model one must look at how these variables act together.
Fluegge computed “amount of added fluoride” different ways and showed models for these. Also a separate model was presented for natural fluoride. Most of the models showed that “amount of added fluoride” was associated with a higher prevalence and incidence of diabetes. In all models, the “number of years a system fluoridated” was associated with a lower prevalence and incidence of diabetes.
The “fluoridation chemical” variable revealed that sodium fluoride was associated with higher prevalence and incidence of diabetes, but fluorosilicic acid and sodium fluorosilicate were associated with a lower prevalence and incidence of diabetes in all models. So while one fluoridation chemical was associated with higher prevalence and incidence of diabetes, this effect would be decreased if the water system was fluoridated for a large number of years.
Several perplexing observations arise when one tries to interpret these results. First, diabetes is a chronic disease and one would expect that prolonged exposure to a hypothesized pathogen would be important. In the Fluegge models, number of years of fluoridation is always associated with lower prevalence and incidence of diabetes. Second, the sensitivity analysis (shown in Table 4) reveals evidence that the variable for “Added fluoride” is problematic. In describing the sensitivity analysis, Fluegge states that 32 counties had natural fluoride levels that were above the optimal level. The computation for the variable “Added fluoride” for these counties was therefore a negative value. The sensitivity analysis removed the data from these 32 counties and is presented in Table 4. The table shows model results for exposure in mg and exposure in ppm. The results are very different for these two approaches to the variable “added fluoride”. For exposure in mg, “added fluoride” is associated with a higher prevalence and incidence of diabetes; for exposure in ppm, “added fluoride” is associated with a lower prevalence and incidence of diabetes. This warrants a closer look at how the variable is computed.
The variable for “added fluoride in mg” is based on county-level water delivery data from the US Geological Survey. Fluegge computed per capita fluoride consumption estimates for each county and the derived values are shown in two histograms (one for 2005 and one for 2010) in the top portion of Figure 5. The histograms are not directly comparable because the scales for the x and y axes are different. The range appears to go from -1 to all the way to 4 mg. How did we get here? Fluegge states that USGS estimates that an individual uses 302.8-378.5 L of water per day. Fluegge reasons that an individual drinks 1.9 L of water daily and this means that “Dividing 1.9L by 302.8 (~0.625%) and 378.5 (=0.5%) liters yields an approximate range of the proportion of the per capita supply that is actually ingested.” Fluegge then goes on to use 0.625 and 0.5 to compute the amount of water that a person drinks, given the USGS water delivery data for the county. This means that a person could be estimated to drink more than 1.9 L per day which seems unlikely, and using estimated consumption level to generate mg of fluoride consumed. As a result, Fluegge is creating a measure that has no validity and inserting it into the GEE model as fluoride exposure. This makes the regression model uninterpretable. Alternatively, the “added fluoride in ppm” variable is simply the level of fluoride in the water system measured as parts per million.
Given all the limitations in the data, the model presented in Table 4 listed as M=2 (exposure in ppm) is the most attractive because it is the most simple. It removes the counties that had natural fluoride levels that exceeded the optimal level and it uses the most straightforward measure of amount of fluoride in a water system. That model does not present a basis for concern that adding fluoride to drinking water is associated with a higher prevalence or incidence of diabetes. If anything, it appears that fluoride in drinking water is associated with a lower prevalence and incidence of diabetes.
Bottom line: Garbage in = Garbage out.
ADDITIONAL NOTES
Biologic basis for hypothesis that fluoride influences incidence and prevalence of diabetes
The literature cited does not establish a compelling argument for a role of fluoride in the pathophysiology of diabetes.
Behavioral Risk Factor Surveillance System (BRFSS) and Diabetes
To have diagnosed diabetes in BRFSS, respondents answer “yes” to the question “Has a doctor ever told you that you have diabetes?” The diagnosis can be subject to recall bias and misinterpretation and does not distinguish between type 1 and type 2. Furthermore, those who have undiagnosed diabetes are included in the “no diabetes” group. As the author discuss in the Introduction, about 30% of diabetes are reportedly undiagnosed.
While BRFSS has produced additional weights to allow small area estimate for metropolitan and micropolitan statistical areas (MMSA), only 153 and 192 MMSAs met the weighting criteria for the 2005 and 2010 data years, respectively.6,7 County-level estimates are reportedly possible using BRFSS data, but caution is needed in the interpretation as data may not well represent small counties with small number of participants.
Potential for compounding misclassification
Note in table 4, that after removing data from 32 counties to do the sensitivity analysis, the number of counties becomes 759 from 887. This is a reduction of 128. If this is not an error in the manuscript, 32 counties are contributing 128 county-year units to the analysis. This highlights another weakness in the design, that is, CDC derived the estimates with assumption that diabetes patterns can be modeled using a few socio-demographic characteristics in a county. If this assumption is weak for a given county, the data for that county are weak and in this analysis each county contributes two observations for each study year (2005 and 2010). So, up to 4 observations could be flawed for each county that does not fit the assumption.
Additives for fluoridation of public water systems
The author does not provide any background or a rationale of examining the types of fluoridation additives as a confounder of relationship between fluoride in drinking water and diabetes. The type of fluoridation additives is usually determined by various engineering/system characteristics such as system and facility size, feed system used, available installation costs etc. As of 2010, 75%, 10% and 15% of US water systems use fluorosilicic acid (FSA: liquid), sodium fluorosilicate (NaFS or Na2SiF6: powder), and sodium fluoride (NaF: powder/crystalline), and 81%, 13% and 7% of population are served by FSA, NaFS, and NaF, respectively.9 Sodium fluoride is most expensive9 and generally used in a small water system only. According to the information provided in the Result section, more than one additive was used in 19% of counties included in the study. Thus this variable is treated as binary variable (i.e. FSA—yes, no) in regression analyses, which means that one fifth of counties in this study represented more than one category in this variable.
The suggestion that added fluoride and also one type of fluoride additive increase risk for diabetes but natural fluoride or other types of fluoride is protective do not make sense, and there is no plausible biological mechanisms to explain them. If fluoride was a contributing factor to diabetes, one would expect a consistent correlation regardless of the particular form of fluoride. The author makes an argument in Discussion that this finding may imply a future policy change to promote the use of FSA rather than NaF for the prevention of diabetes and the potential cost saving. However, sodium fluoride is the least common fluoridation additive and is used mostly in small US communities. Thus even if this association was true, the anticipated cost-saving from banning NaF would be limited.
DISCLAIMER
The findings and conclusions on this page are those of the Fluoride Science Editorial Board and do not necessarily represent those of AAPHD. These reviews are not mandates for compliance or spending. Instead, they provide information and options for decision makers and stakeholders to consider when determining which programs, services, and policies best meet the needs, preferences, available resources, and constraints of their constituents.
Document last updated December 16, 2016
REFERENCES
- Urbansky ET. Fate of fluorosilicate drinking water additives. Chem Rev. 2002;102(8):2837-54
- Maguire A, Zohouri FV, Mathers JC et al. Bioavailability of fluoride in drinking water: a human experimental study. J Dent Res. 2005;84(11):989-93
- Whitford GM, Sampaio FC, Pinto CS et al. Pharmacokinetics of ingested fluoride: lack of effect of chemical compound. Arch Oral Biol. 2008;53(11):1037-41
- McClure FJ. Availability of fluorine in sodium fluoride vs. sodium fluosilicate. Public Health Rep. 1950;65(37):1175-86
- Zipkin I, McClure FJ. Complex fluorides: caries reduction and fluorine retention in the bones and teeth of white rats. Public Health Rep. 1951;66(47):1523-32
- Centers for Disease Control and Prevention. Behavioral Risk Factor Surveillance System Summary Data Quality Report. August 25, 2006. Available at http://www.cdc.gov/brfss/annual_data/2005/pdf/2005summarydataqualityreport.pdf
- Centers for Disease Control and Prevention. Behavioral Risk Factor Surveillance System. 2010 Summary Data Quality Report. Revised: May 2, 2011. Available at http://www.cdc.gov/brfss/annual_data/2010/pdf/2010_summary_data_quality_report.pdf
- Center for Disease Control and Prevention. Methodology for County-Level Estimates. Available at https://www.cdc.gov/diabetes/atlas/obesityrisk/County_Methods.html
- Centers for Disease Control and Prevention. Water Fluoridation Principles and Practices. Water Fluoridation Additives. Atlanta, GA. 2015.